攻克人类医学难关:医施德AI云诊科研团队在癌症早筛领域的重大突破
- 2024-08-15 13:46
- 网络
在医学领域,癌症一直是人类面临的一大挑战。医施德科研团队在癌症早筛领域取得了突破性进展,其AI技术已全面覆盖人类致死率排名前50的肿瘤类型,包括胰腺癌、食道癌、胃癌、肺癌、肝癌、胆囊癌、乳腺癌、宫颈癌、甲状腺癌、结直肠癌、脑肿瘤、鼻咽癌、肾肿瘤、肾上腺肿瘤、骨肉肿瘤、骨髓肿瘤等,这一突破不仅为癌症患者带来了福音,也为全球癌症防控事业贡献了重要力量,将AI科技为人类社会改变医疗健康的未来。
癌症发展的阶段与早期诊断的挑战
癌症的发展经历了从正常细胞到原位癌、早期癌、浸润癌直至转移癌的多个阶段。早期诊断对于癌症的治疗和预后至关重要。然而,传统的CT、核磁、病理等影像学检查手段在癌症早期诊断中存在诸多局限性,如无法检测到1万-50万细胞病变、基因突变期无法识别等问题。
医施德-AI云诊系统在肿瘤诊断领域的突破性优势
在肿瘤诊断领域展现出六大突破性优势:
肿瘤癌症原发定位:准确判断肿瘤的原发部位,为治疗提供有力依据。
早始期分辩良性与恶性肿瘤:在癌症发展的早期阶段,就能区分良性和恶性肿瘤。
高敏感度病变识别:能够识别出1万-50万细胞病变,远超过传统检查方法的敏感度。
监测肿瘤发展状态:持续跟踪肿瘤的发展情况,为治疗提供实时反馈。
高可及性:不受医疗条件、空间和时间限制,使更多患者能够享受到先进的AI诊断服务。
AI规模化诊断识别:能够高效、准确地识别大量病例中的潜在癌症患者。
人工智能诊断技术的核心算法与优势
医施德AI云诊系统集成了多种先进的人工智能算法,包括线性回归、逻辑回归、决策树、神经网络、复值神经⽹络、馈神经⽹络、循环神经⽹络、⾃然语⾔处理NLP、图像分割、进化算法、遗传算法GA、粒⼦群优化PSO、卷积神经⽹络CNN、 SVM、朴素⻉叶斯等。这些算法能够处理和分析海量的医疗数据,为医生提供准确的诊断结果和个性化的治疗方案。通过应用这些算法,AI云诊系统不仅提高了诊断的准确性和效率,还能够帮助医生制定更个性化的治疗方案,预测疾病风险,改善病患体验,并促进远程医疗的发展。
AI诊断技术给人类带来的利益
1、提高诊断准确性:人工智能算法可以分析大量的医疗数据,包括影像资料、基因信息、病历记录等,从而更准确地识别疾病模式,减少人为错误和主观判断的影响。这有助于确保病患得到更准确的诊断,为治疗提供更有针对性的方案。
2、提高诊断效率:传统的医疗诊断过程可能需要耗费医生大量的时间和精力。而人工智能可以在短时间内分析大量数据,提供快速的诊断结果。这不仅可以减轻医生的工作负担,还可以使病患更快地得到诊断和治疗,从而缩短病程,提高治疗效果。
3、辅助个性化治疗:通过对病患的病史、基因信息、生活习惯等数据进行分析,人工智能可以帮助医生制定更个性化的治疗方案。这种个性化治疗可以更好地满足病患的需求,提高治疗效果,减少不必要的副作用。
4、精准预测疾病风险:人工智能可以通过分析病患的健康数据,精准预测其未来可能面临的健康风险。这有助于病患提前采取预防措施,降低疾病发生率,提高生活质量。
5、改善病患体验:人工智能技术可以优化医疗流程,使病患在就医过程中更加便捷、舒适。例如,通过智能导诊系统,病患可以更快地找到需要的科室和医生;通过在线问诊平台,病患可以在家中就能得到专业的医疗建议和指导。
6、促进远程医疗发展:人工智能诊断技术使得远程医疗成为可能。通过远程传输医疗影像和病历数据,医生可以在异地为病患提供诊断和治疗建议。这有助于缓解医疗资源分布不均的问题,使更多地区的病患能够享受到优质的医疗服务。
医施德AI云诊科研团队
医施德AI云诊科研团队由国内外数百名专家、学者和研究员组成,团队成员来自哈佛大学、麻省理工学院、斯坦福大学等世界知名高等院校。他们凭借深厚的学术背景和丰富的实践经验,共同推动了AI在癌症早筛领域的创新发展。医施德AI云诊系统的成功应用,不仅体现了团队在人工智能领域的卓越实力,也彰显了他们在医学领域的深厚造诣和人文关怀。
医施德AI云诊科研团队在癌症早筛领域的重大突破,为全球癌症防控事业注入了新的活力。未来,随着人工智能技术的不断发展和应用,我们有理由相信,癌症将不再是很难发现和不治之症,更多患者将受益于先进的医学技术和科研成果。
参考论文
1. Sung, H. et al. Global cancer statistics 2020: GLOBOCAN estimates of incidence and mortality worldwide for 36 cancers in 185 countries. CA Cancer J Clin. https://doi.org/10.3322/caac.21660 (2021).
2. Sawaya, G. F., Smith-McCune, K. & Kuppermann, M. Cervical cancer screening: more choices in 2019. JAMA 321, 2018–2019 (2019).
3. Fremont-Smith, M., Marino, J., Griffin, B., Spencer, L. & Bolick, D. Comparison of the SurePath liquid-based Papanicolaou smear with the conventional Papanicolaou smear in a multisite direct-to-vial study. Cancer Cytopathology. 102, 269–279 (2004).
4. Chen, W. et al. Cancer statistics in China, 2015. CA Cancer J. Clin. 66, 115–132 (2016).
5. Nayar, R. & Wilbur, D. C. The Pap Test and Bethesda 2014. Acta Cytol. 59, 121–132 (2015).
6. de Grey, A. D. Artificial intelligence and medical research: time to aim higher? Rejuvenation Res. 19, 105–106 (2016).
7. Attia, Z. I. et al. Screening for cardiac contractile dysfunction using an artificial intelligence-enabled electrocardiogram. Nat. Med. 25, 70–74 (2019).
8. Esteva, A. et al. Dermatologist-level classification of skin cancer with deep neural networks. Nature 542, 115–118 (2017).
9. Madabhushi, A. & Lee, G. Image analysis and machine learning in digital pathology: challenges and opportunities. Med. Image Anal. 33, 170–175 (2016).
10. Krizhevsky, A., Sutskever, I. & Hinton, G. ImageNet classification with deep convolutional neural networks. Commun. Acm. 60, 84–90 (2017).
11. Hou, L. et al. Sparse autoencoder for unsupervised nucleus detection and representation in histopathology images. Pattern Recognit. 86, 188–200 (2019).
12. Li, C., Wang, X., Liu, W. & Latecki, L. J. DeepMitosis: mitosis detection via deep detection, verification and segmentation networks. Med. Image Anal. 45, 121–133 (2018).
13. Hu, B. et al. Unsupervised learning for cell-level visual representation in histopathology images with generative adversarial networks. IEEE J. Biomed. Health Inform. 23, 1316–1328 (2019).
14. Sornapudi, S. et al. Deep learning nuclei detection in digitized histology images by superpixels. J. Pathol. Inform. 9, 5 (2018).
15. Xu, J., Luo, X., Wang, G., Gilmore, H. & Madabhushi, A. A Deep Convolutional Neural Network for segmenting and classifying epithelial and stromal regions in histopathological images. Neurocomputing 191, 214–223 (2016).
16. Chen, H. et al. Mitosis detection in breast cancer histology images via deep cascaded networks. In Proc. of the Thirtieth AAAI Conference on Artificial Intelligence. 30, 1160–1166 (2016).
17. Sirinukunwattana, K. et al. Locality sensitive deep learning for detection and classification of nuclei in routine colon cancer histology images. IEEE Trans. Med Imaging 35, 1196–1206 (2016).
18. Campanella, G. et al. Clinical-grade computational pathology using weakly supervised deep learning on whole slide images. Nat. Med. 25, 1301–1309 (2019).
19. Coudray, N. et al. Classification and mutation prediction from non-small cell lung cancer histopathology images using deep learning. Nat. Med. 24, 1559–1567 (2018).
20. Skrede, O. J. et al. Deep learning for prediction of colorectal cancer outcome: a discovery and validation study. Lancet 395, 350–360 (2020).
论文来源
(1)https://www.nature.com
(2)https://www.springer.com
本文地址:http://www.qqylw.com.cn/xinwen/2240.html
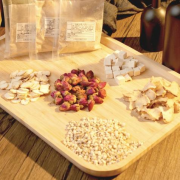
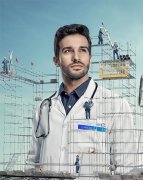
品牌策划
BRAND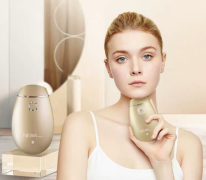
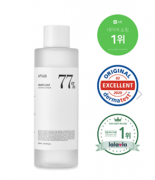
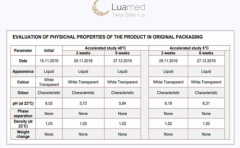